Program
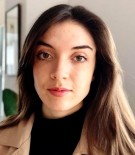
Consultant Health Economist at Hardian Health, UK
With infinite demands for healthcare and limited resources and increasing costs to meet them, the need for novel technologies to demonstrate value beyond safety and clinical efficacy is more than ever. Simultaneously, healthcare professionals, payers and patients require sufficient information to support decision making around the use of AI in care pathways.
This session will provide a brief introduction to the field of health economics and outcomes research (HEOR). Attendees can expect an overview of the HEOR methods and perspectives relevant to the adoption of AI across healthcare markets, such as budget impact modelling and cost-effectiveness analysis. An application of AI in radiology will also be showcased to provide technology developers, industry and policymakers an example of when health economic evidence should be considered and the requirements, and methods needed to generate evidence of value and affordability.
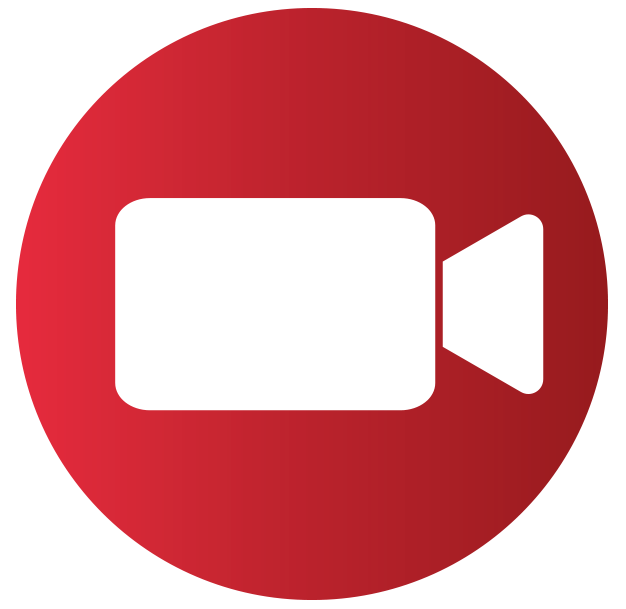
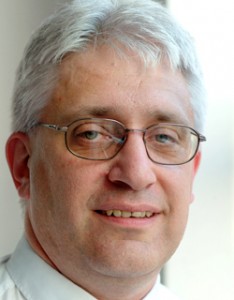
Founder and General Manager of the Belgian e-Health Platform, CEO of Smals, Belgium
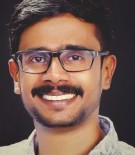
Head of Product and Technology, SkinVision, Netherlands
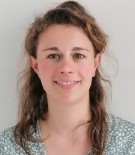
ML Specialist, SkinVision, Netherlands
As the global incidence of skin cancer continues to rise, the increasing number of diagnoses is putting immense pressure on healthcare systems. SkinVision empowers individuals by allowing them to examine skin spots using their mobile phones, encouraging early detection of skin cancer and ensuring timely medical intervention for those in need.
In this talk, we will explore what SkinVision is, how it works, our role in the care pathway, and the challenges we face along the way.
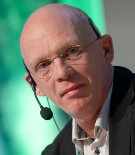
Trusted AI Labs (TRAIL) and Director of PiLAB, UCLouvain, Belgium
Continual active learning is a transformative approach in the enhancement of screening techniques, particularly for breast cancer, colon cancer, and certain neurological diseases. This method integrates continuous learning and active data collection, allowing artificial intelligence (AI) systems to progressively improve their accuracy and efficiency. Drawing inspiration from the MyPeBS (My Personal Breast Screening) project, which focuses on personalized breast cancer screening across Europe, continual active learning involves AI systems that actively query experts in uncertain cases, learning from these interactions to refine their predictive models over time. This iterative process not only enhances the AI’s diagnostic capabilities but also ensures that it adapts to new data and evolving medical knowledge, leading to more accurate and timely detection of diseases. By leveraging continual active learning, healthcare providers can significantly improve the effectiveness of screening programs, ultimately leading to earlier detection and better patient outcomes.
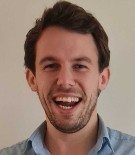
Technical Physician, The Netherlands Cancer Institute, NKI, Netherlands
“In oncology, radiologists report the response of a patient undergoing anticancer treatment by measuring diameters of the tumor on medical imaging. This has been shown to yield substantial interobserver variability and correlates poorly with the overall survival of the patient. Measuring the total tumor volume in 3D CT scans is more accurate but not clinically feasible due to its time-consuming nature. Here, AI models have been developed to measure the entire tumor volume precisely and automatically, which enables total tumor volume follow-up.
In this talk, we will explore how the Netherlands Cancer Institute is developing these models, and even more importantly, have implement such models with the aim to improve the quality of care provided to patients. We will cover all relevant topics in the lifecycle, from the clinical problem that needs to be solved to the digital infrastructure needed to deploy these models, with one overall goal: provide accurate information on the response to treatment so the patient and physician can make better informed decisions.”
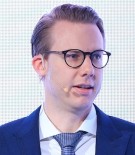
Partner at Syte, Germany
• Success factors for an increased AI implementation in healthcare
• Description of a large scale real world AI in Diabetes and Obesity usecase with >150.000 patients
• Benchmarking between AI and physician decision making
• AI driven efficiency increase for GLP 1 Receptor Agonists
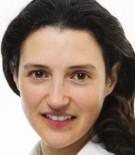
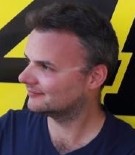
Chief Innovation Officer, HUmani University Hospital Charleroi-Chimay, Belgium
This talk will present the value proposition of the TEF-Health project, showcasing both the consortium’s and an SME’s perspectives on introducing an AI healthcare solution to the European market. It will highlight the importance of timing—both in terms of TEF-Health’s strategic timing and the urgency for startups navigating market entry.
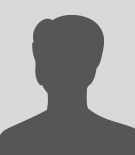
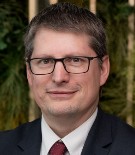
Professor at University Hospital (CHU) Reims and Director of Institut d’Intelligence Artificielle en Santé, University of Reims Champagne-Ardenne, France
This lecture will explore the transformative potential of GenAI in healthcare, highlighting its journey from technological development to real-world application and value creation. We will delve into latest advancements in generative AI, focusing on how these technologies, such as RAG, are being applied to enhance clinical workflow, medical research, and patient care.
Key discussion will include the development of AI model based on real ground health data, ethical and regulatory challenges, and the integration of these innovations into existing healthcare systems. By examining both the technical and practical aspects, we aim to provide insights into how healthcare organizations can harness the power of AI to drive innovation, improve patient outcomes, and create sustainable value.
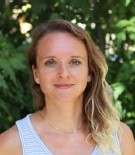
Medical Writer at Staburo, Germany
This talk delves into the use of generative artificial intelligence in medical writing, particularly focusing on the creation of Plain Language Summaries (PLS) for clinical trials. Plain Language Summaries (PLS) are simplified versions of clinical trial results designed to be easily understood by non-experts, including patients, study participants and public, by providing transparency and accessibility. With the increasing demand for transparency in clinical research, PLS have become a regulatory requirement under the European Union Clinical Trials Regulation. AI has the potential to streamline the process of generating these summaries, producing large volumes of text quickly. However, this raises critical questions about the quality of AI-generated summaries, especially when it comes to accurately interpreting complex study results and maintaining the clarity needed for non-expert audiences.
In this session, we will explore findings from a study that compares AI-created PLS with those written by experienced medical writers. Key aspects such as correctness, completeness, and comprehensibility will be examined. We will also discuss whether AI can truly match human-written summaries in quality, or if the best approach lies in a hybrid model, where AI assists human writers to enhance efficiency without compromising accuracy.
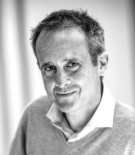
Chief Innovation Officer at MyData-TRUST, Belgium
This talk explores how generative AI can be harnessed to meet the specific needs of data protection in the life sciences sector. Instead of focusing on AI development, we will dive into three practical use cases that highlight MyData-TRUST’s enhanced efficiency through AI-driven solutions:
- Regulatory Queries: Streamlining responses to complex regulatory inquiries.
- Document Queries: Enhancing search capabilities within extensive document repositories.
- Document Compliance Checks: Automating compliance assessments to ensure adherence to regulatory standards.
These examples illustrate how generative AI can revolutionize data protection practices, making processes faster, more accurate, and compliant with industry regulations.
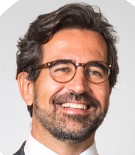
CEO, Quinten, France
- Cohort simulation methods have been successfully used for many years in regulatory and HTA decision contexts to compensate clinical trial’s intrinsic limitations in time, diversity and comparators. However, the use of these methods remains seldom, based on ad-hoc models.
- Such AI-driven cohort simulation methods are the only way forward to reduce uncertainties about the long-term impact of therapeutic innovations, and to provide objective decision support in a context of ever-increasing risk-aversion and need for cost-efficiency
- Disease-centric cohort simulators, developed and duly validated in transparency with regulators and HTAs, based on high-quality Real-World Data sources and AI allows for de-risking and accelerating therapeutic innovations and the rise of personalized medicine.
Cohort simulation methods, underused but vital in regulatory and HTA decisions, compensate for clinical trial limitations in time, diversity and comparators. They mitigate uncertainties about long-term therapeutic impacts, essential in risk-averse, cost-efficient contexts. AI powered disease-centric simulators, validated transparently, based on Real-World Data, aid in de-risking and accelerating personalized medicine innovations.
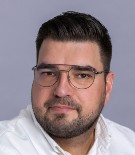
Medical Physicist-Clinical Data Scientist, Postdoc at MAASTRO clinic-Maastricht University, Netherlands
In my presentation I will explore the critical role of AI in transforming the landscape of health data management across Europe. Drawing from my extensive experience as a researcher in AI and FAIR data infrastructures, as well as my involvement in various international research projects, I will present how AI-driven solutions can revolutionize the curation, integration, and reuse of personal health data. This presentation will highlight the challenges of fragmented health data, the potential of AI to automate data curation processes, and the importance of creating interoperable and patient-centric health records. By showcasing real-world examples and use cases, I aim to demonstrate how these advancements can empower patients and enhance clinical research.
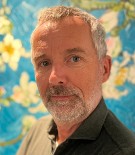
Chairman of the Board of Yuma, Netherlands
More and more, people start to appreciate the importance and value of data. This is not only accelerated by the ongoing growth and adoption of AI, but also because it is one of the cornerstones of the EU data-strategy. More specifically, the EU data-strategy also encourages to increase the potential of data by supporting data sharing approaches using ‘data spaces’. In his talk Hans will high-light what data spaces are and how they relate to working with health-related data in the EU. He will use a number of examples from his own experience to describe the current state-of-practice and identify challenges and opportunities going forward.
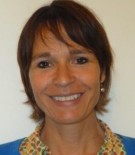
Scientific Director (A.I.) Data Governance at Sciensano, Belgium and Professor in Smart Statistics for Policy Design and Development at Maastricht University, the Netherlands
AI offers a lot of potential for health and health demand forecasting. The prerequisites for AI are Findable Accessible Interoperable and Reusable data or in other words data that are FAIR. Data at most public health institutes are not (yet) FAIR. Sciensano started a data strategy and implementation plan in 2021 from which evolved the creation of a scientific directorate Data Governance in 2024.
This talk will elaborate on the realities of data at public health institutes and its current data governance plans such as an AI cell and charter. Using my previous professional experiences at 2 bureaus of official statistics, I also will elaborate on examples in official statistics that show the power of structured data (the use of registers), their potential for forecasting and the recognition of ‘public statistical’ output as a leverage for accessing data and data quality by complying to the code of practise in official statistics.
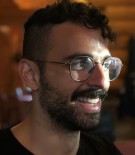
Postdoctoral Fellow at the Ernst Srüngmann Institute for Neuroscience, Germany
Large Language Models (LLMs) have become central to advancing automation and decision-making across various sectors, raising significant ethical questions. This talk is based on a recent research project in which we proposed a comprehensive comparative analysis of the most advanced LLMs to assess their moral profiles. We subjected several state-of-the-art models to a selection of ethical dilemmas and found that all the proprietary ones are mostly utilitarian and all of the open-weights ones align mostly with values-based ethics. Furthermore, when using the Moral Foundations Questionnaire, all models we probed – except for one – displayed a strong liberal bias. Lastly, we causally intervened in one of the studied models and were able to reliably steer the model’s moral compass to showcase different ethical preferences. All of these results suggest that there is an implicit ethical dimension in already deployed LLMs, an aspect that is generally overlooked.
The fact that current LLMs have an implicitly learned moral compass is of particular relevance in critically sensitive contexts, such as in healthcare. I will lay out my forecasts on how the patient-care provider relationship might be altered in the near term and what consequences these findings might have for public health.
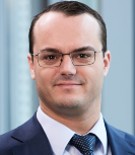
Partner, Hogan Lovells, Belgium
The purpose of the presentation will be to give an overview of the regulatory requirements and challenges which apply to manufacturers of AI-based medical devices in the EU. The presentation will start with a discussion of current key considerations for the CE marking of these devices under the EU MDR and IVDR before analysing the impact and challenges posed by the AI Act on future conformity assessments; The presentation will also include some considerations from a healthcare professional perspective on the use of AI-based medical devices in daily practices.
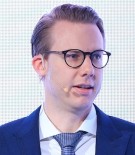
Partner at Syte, Germany
• The EU and US AI Act
• Differences in their implementation for AI in healthcare
• Potential pathways to measure medical and economic impact of AI in healthcare
• Ethical Guidelines in AI-driven healthcare decision-making
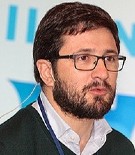
Innovation Director at Bahia Software, Spain
Developing AI systems for use in medical settings is a complex, multi-stage process. Relatively few AI developers publish the methodology of such developments. While healthcare organisations and physicians see many approved devices without sufficient evidence, startups and companies developing AI systems are demanding technical, operational and ethical standards. Meanwhile, regulators such as the US Food and Drug Administration (FDA) or the European Medicines Agency (EMA) have approved hundreds of AI-powered systems for use in hospitals and clinics through less rigorous processes than those for drugs.
It has become clear to policymakers, regulators, clinicians, patients and the scientific and technological community that AI standardisation should be implemented to ensure patient rights, fundamental values and the desired impact on healthcare organisations. The presentation aims to share the CHAIMELEON’s findings in this specific topic. A preliminary toolkit with guidelines related to the design, development and validation of AI systems in healthcare will be shared with the audience. Finally, some concrete examples of AI models in the health domain will be presented.
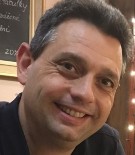
Chief Information Officer at General Hospital of Granollers/ Barcelona, Spain
When you realize that we are witnessing a revolution rather than just a new technological advancement, you have an obligation to do everything in your power to integrate it into your organization.
This presentation will outline the roadmap for incorporating Generative Artificial Intelligence at Hospital General de Granollers. It will also include real-world use cases currently being developed and implemented. Attendees will gain insights into the strategic approach taken by the hospital, the challenges faced, and the transformative impact of this cutting-edge technology on healthcare practices.
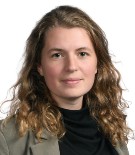
Researcher at TU Eindhoven (process analytics) and St. Antonius Hospital, Netherlands
The discharge process is a critical bottleneck in patient care, often leading to longer hospital stays when aftercare is not arranged in time. In this talk, I will discuss the challenges faced in predicting both discharge dates and necessary follow-up care using AI. I will share insights and solutions encountered throughout my research journey, highlighting practical applications and future directions.
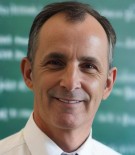
Healthcare professionals worldwide face a significant administrative burden, spending a large portion of their time on documentation rather than patient care. Studies indicate that, on average, over 50% of a clinician’s time is taken up by bureaucratic tasks such as clinical records, discharge notes, and referrals. This not only impacts the quality of care but also contributes to provider burnout, reduced job satisfaction, and compromised patient experience. The inefficiency created by these tasks leads to high levels of stress among healthcare workers and, ultimately, limits the amount of quality time they can dedicate to patients.
In this talk I will present how AI-powered solutions can tackle the documentation burden, allowing clinicians to refocus on patient care. By leveraging natural language processing and voice recognition technologies, AI tools can capture and transcribe consultations, automatically generating accurate medical notes, discharge summaries, and referrals. This technology enables clinicians to produce high-quality, standardized records with minimal effort, saving valuable time and reducing the risk of burnout. Some solutions integrate seamlessly with existing electronic health record systems, enhancing workflow without disrupting familiar routines. Collectively, these AI advancements not only improve efficiency in clinical settings but also enhance patient-provider interactions, allowing healthcare professionals to focus more fully on patient care ultimately improving satisfaction on both sides of the consultation.
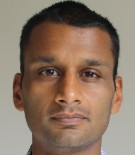
Advisor at the World Health Organization (WHO), France
The World Health Organization has prioritized artificial intelligence (AI) as a technology that could assist governments meet global commitments to achieve universal health coverage. Yet AI also raises numerous trans-national, ethical, legal, commercial and social concerns that pose risks to patients, providers, health systems, and societies. In response, the WHO convened an expert group on the ethics and governance of artificial intelligence in health in 2019. Over the last five years, the Expert Group has issued international guidance that identifies the risks and challenges for the use of AI, ethical principles that should underpin the design, development and use of AI, and models of governance to assure its appropriate use. This includes additional guidance on the use of generative AI (large multi-modal models in health care), the use of AI for pharmaceutical development and delivery, and the relationship of AI to ageism. Currently, WHO is examining how research ethics can provide effective oversight of AI-related health research, while also providing support to Member States, providers, and developers to integrate the principles and recommendations from WHO into their on-going use of AI.